Unified Embedding and Metric Learning for Zero-exemplar Event Detection

@inproceedings{hussein2017unified, title = {Unified Embedding and Metric Learning for Zero-Exemplar Event Detection}, author = {Hussein, Noureldien and Gavves, Efstratios and Smeulders, Arnold WM}, booktitle = {Proceedings of the IEEE Conference on Computer Vision and Pattern Recognition}, year = {2017} }
Abstract
Problem
Event detection in unconstrained videos is conceived as a content-based video retrieval with two modalities: textual and visual. Given a text describing a novel event, the goal is to rank related videos accordingly. This task is zero-exemplar, no video examples are given to the novel event.
MethodRelated works train a bank of concept detectors on external data sources. These detectors predict confidence scores for test videos, which are ranked and retrieved accordingly. In contrast, we learn a joint space in which the visual and textual representations are embedded. The space casts a novel event as a probability of pre-defined events. Also, it learns to measure the distance between an event and its related videos.
ResultsOur model is trained end-to-end on publicly available EventNet. When applied to TRECVID Multimedia Event Detection dataset, it outperforms the state-of-the-art by a considerable margin.
Method
We pose the problem of zero-exemplar event detection as learning from a repository of pre-defined events. Given video exemplars of events “removing drywall” or “fit wall times”, one may detect a novel event “renovate home” as a probability distribution over the predefined events.
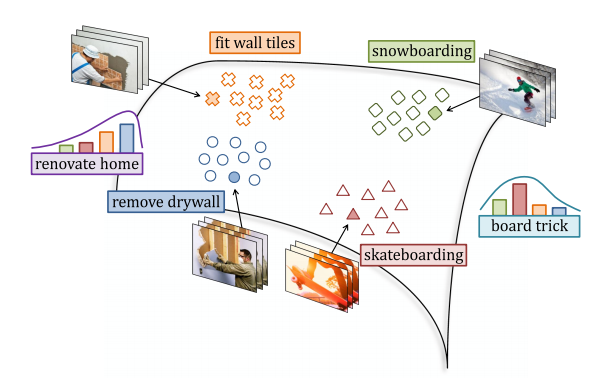
Three families of methods for zero-exemplar event detection: (a), (b) and (c). They build on top of feature representations learned a priori (i.e. initial representations), such as CNN features x for a video v or word2vec features y for event text query t. In a post-processing step, the distance \theta is measured between the embedded features. In contrast, our model rather falls in a new family, depicted in (d), for it learns unified embedding with metric loss using single data source.
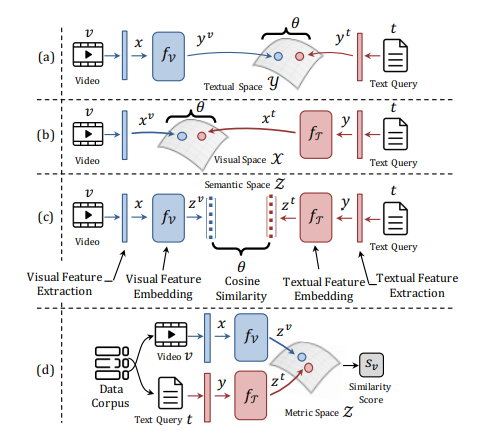
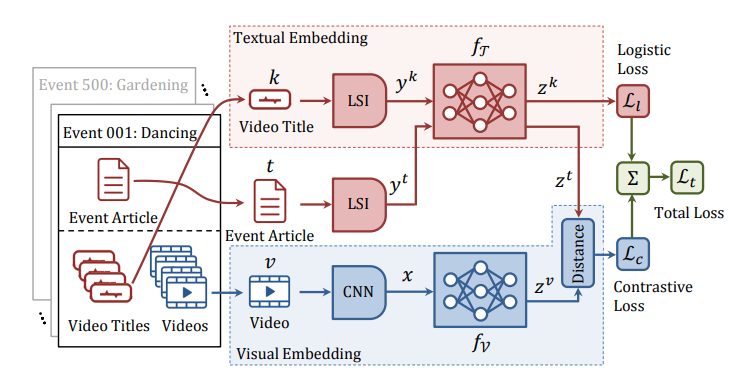
Model overview. Using dataset \mathcal{D}^z of M event categories and N videos. Each event has a text article and a few videos. Given a video x with text title k, belonging to an event with article t, we extract features x, y^k, y^t respectively. At the top, network f_{\mathcal{T}} learns to classify the title featurey^k into one of M event categories. In the middle, we borrow the network f_{\mathcal{T}} to embed the event article’s feature y^t as z^t \in \mathcal{Z}. Then, at the bottom, the network f_{\mathcal{V}} learns to embed the video feature x as z^v \in \mathcal{Z} such that the distance between (z^v, z^t) is minimized, in the learned metric space \mathcal{Z}.
Results
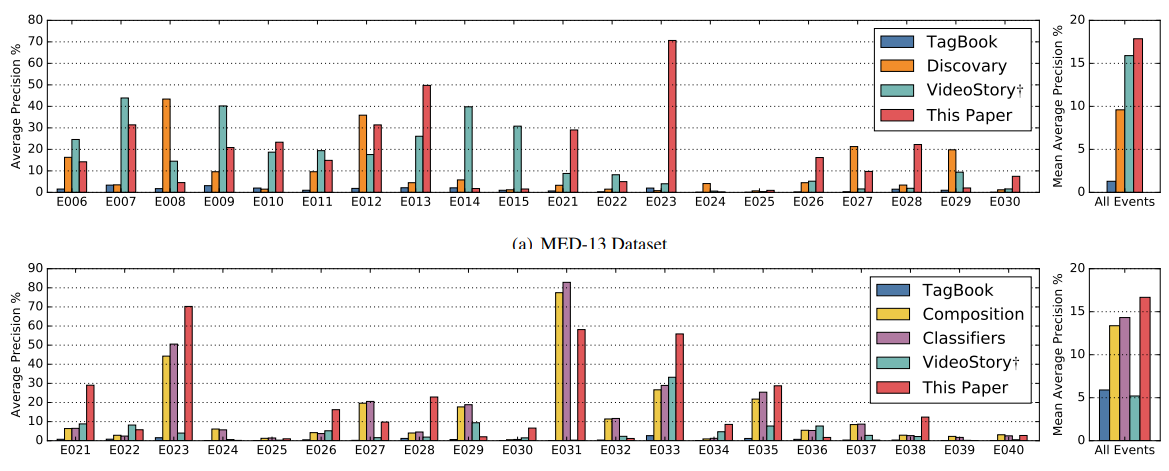
Event detection accuracies: per-event average precision (AP %) and per-dataset mean average precision (mAP%) for MED-13 and MED-14 datasets. We compare our results against TagBook, Discovary, Composition, Classifiers and VideoStory.
Method | MED-13 | MED-14 |
---|---|---|
TagBook | 12.90 | 05.90 |
Discovary | 09.60 | ------- |
Composition | 12.64 | 13.37 |
Classifiers | 13.46 | 14.32 |
VideoStory | 15.90 | 05.20 |
This Work | 17.86 | 16.67 |
Performance comparison between our model and related works. We report the mean average precision (mAP %) for MED13 and MED-14 datasets.