VideoGraph: Recognizing Minutes-Long Human Activities in Videos

@inproceedings{hussein2019videograph, title = {VideoGraph: Recognizing Minutes-Long Human Activities in Videos}, author = {Hussein, Noureldien and Gavves, Efstratios and Smeulders, Arnold WM}, booktitle = {ICCV Workshop on Scene Graph Representation and Learning}, year = {2019} }
Abstract
Problem
Many human activities take minutes to unfold. To represent them, related works opt for statistical pooling, which neglects the temporal structure. Others opt for convolutional methods, as CNN and Non-Local. While successful in learning temporal concepts, they fall short of modeling minutes-long temporal dependencies.
MethodWe propose VideoGraph, a method to achieve the best of two worlds: represent minutes-long human activities and learn their underlying temporal structure. VideoGraph learns an undirected graph representation for human activities. The graph, its nodes and edges are learned entirely from video datasets, making VideoGraph applicable to video understanding tasks without node-level annotation.
ResultsThe result is improvements over related works on benchmarks: Breakfast, Epic-Kitchen and Charades. Besides, we demonstrate that VideoGraph is able to learn the temporal structure of human activities in minutes-long videos
Method
Figure 1: The activity of “preparing coffee” can be represented as undirected graph of unit-actions. The graph is capable of portraying the many ways one can carry out such activity. More over, it preserves the temporal structure of the unit-actions. Reproduced from.
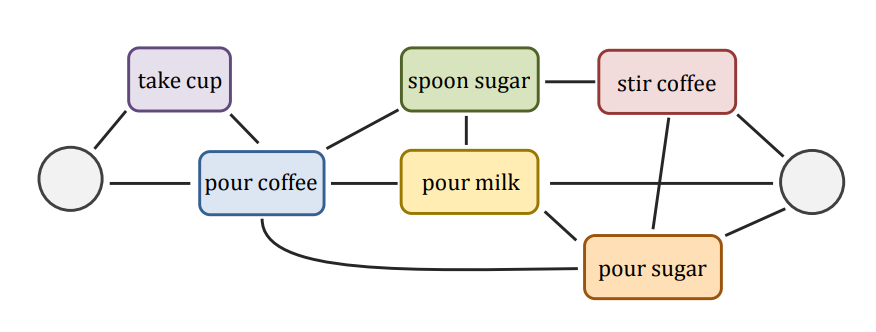
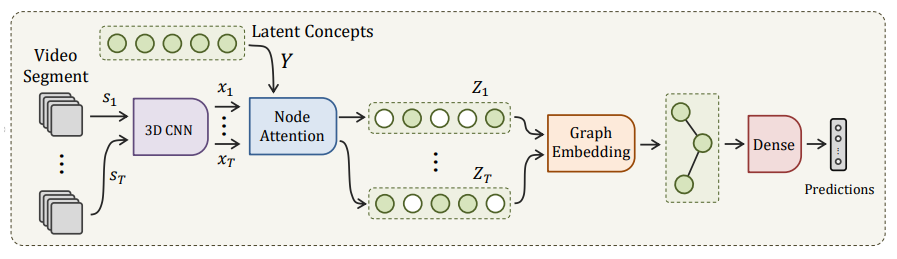
Overview diagram of the proposed VideoGraph. It takes as input a video segment s_i of 8 frames from an activity video v. Then, it represents it using standard 3D CNN, e.g. I3D. The corresponding feature representation is x_i. Then, a node attention block attends to a a set of N latent concepts based on their similarities with x_i, which results in the node-attenative representation Z_i. A novel graph embedding layer then processes Z_i to learn the relationships between its latent concepts, and arrives at the final video-level representation. Finally, an MLP is used for classification.
(a) Node attention block measures similarities \alpha between segment feature x_i and learned nodes \hat{Y}. Then, it attends to each node in \hat{Y} using \alpha. The result is the node-attentive feature Z_i expressing how similar each node to x_i. (b) Graph Embedding layer models a set of T successive node-attentive features Z using 3 types of convolutions. i. Timewise Conv1D learns the temporal transition between node-attentive features \{Z_i, ..., Z_{i+t}\}. ii. Nodewise Conv1D learns the relationships between nodes \{z_{i,j}, ..., z_{i,j+n}\}. iii. Channelwise Conv3D updates the representation for each node z_{ij}.
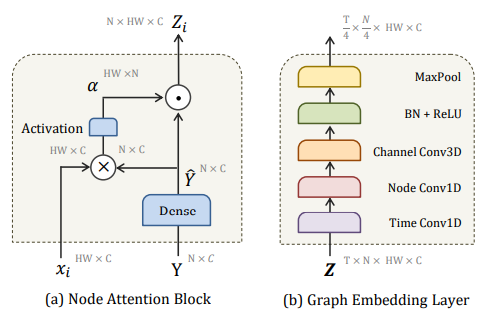
(a) Timewise Conv1D learns the temporal transition between successive nodes-embeddings \{Z_i, ..., Z_{i+t}\} using kernel k^T of kernel size t. (b) Nodewise Conv1D learns the relationships between consecutive nodes \{z_{i,j}, ..., z_{i,j+n}\} using kernel k^N of kernel size n.
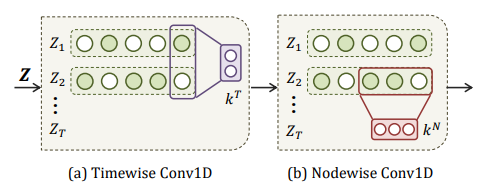
Results
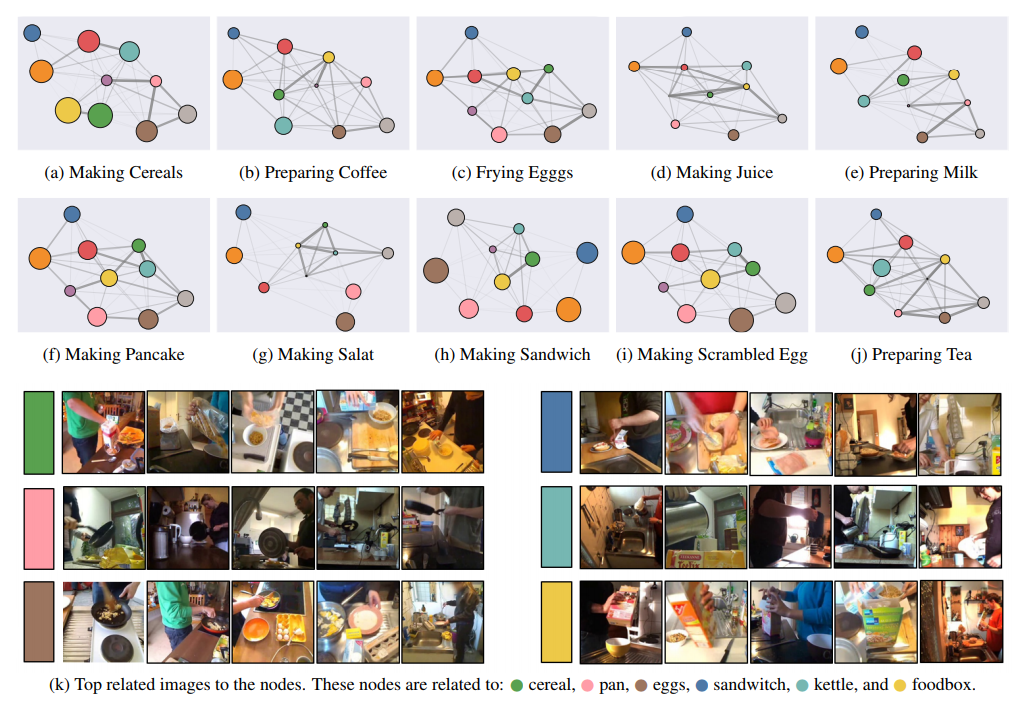
We visualize the relationship discovered by the first layer of graph embedding. Each sub-figure is related to one of the 10 activities in Breafast dataset. In each graph, the nodes represent the latent concepts learned by graph-attention block. Node size reflects how how dominant the concept, while graph edges emphasize the relationship between the nodes.
Method | Breakfast Acc. (%) | Breakfast mAP (%) | EPIC-Kitchens mAP (%) |
---|---|---|---|
ResNet-152 | 41.13 | 32.65 | ------- |
ResNet-152 + ActionVLAD | 55.49 | 47.12 | ------- |
ResNet-152 + Timeception | 57.75 | 48.47 | ------- |
ResNet-152 + VideoGraph | 59.12 | 49.38 | ------- |
I3D-152 | 58.61 | 47.05 | 48.86 |
I3D-152 + ActionVLAD | 65.48 | 60.20 | 51.45 |
I3D-152 + Timeception | 67.07 | 61.82 | 55.46 |
I3D-152 + VideoGraph | 69.45 | 63.14 | 55.32 |
VideoGraph outperforms related works using the same backbone CNN. We experiment 2 different backbones: I3D and ResNet-152. We experiment on two different tasks of Breakfast: activity classification and unit-action multi-label classification. And for Epic-Kitchen, we experiment on the multi-label classification.